Generative AI is being hailed as the most transformative technology of the decade, with the potential to change how organizations operate. Yet its impact across the enterprise has been underwhelming. Consider how many roles in your organization have been profoundly altered by AI in the past year. Likely, the answer is few.
Technology experts share this skepticism. MIT’s Daron Acemoglu, for example, forecasts that AI will touch less than 5% of tasks over the next 10 years, and only increase US productivity by 0.5% and GDP growth by 0.9%. This contrasts sharply with the belief that generative AI will transform the workplace, an idea that gained momentum in late 2022 when ChatGPT first became publicly available.
Despite the gulf between expectation and reality, investment in AI remains strong. 73% of U.S. companies have adopted AI in some area of the business, and the generative AI market is expected to reach $1.3 trillion by 2032.
Enterprises are also heavily investing in AI copilots, smart assistants that support decision-making by automating manual tasks, often positioned as critical to tapping into AI's potential. Forbes reported that 51% of companies are adopting AI for process automation and Microsoft recently shared that over 77,000 organizations use their AI copilot.
Despite these investments, enterprises face big challenges in terms of adoption and impact. In this article, we’ll look at the barriers to reaching AI’s true potential and explore the technologies needed to remove them.
Why do security teams need copilots?
It’s easy to see why AI copilots are viewed as the next essential tool for security teams. They promise to improve efficiency, enhance interoperability across systems, and reduce incident response times.
The efficiency gains are particularly appealing; by automating routine tasks, security teams can free up time to focus on more complex issues. But the question remains the same: can AI copilots actually deliver on these promises?
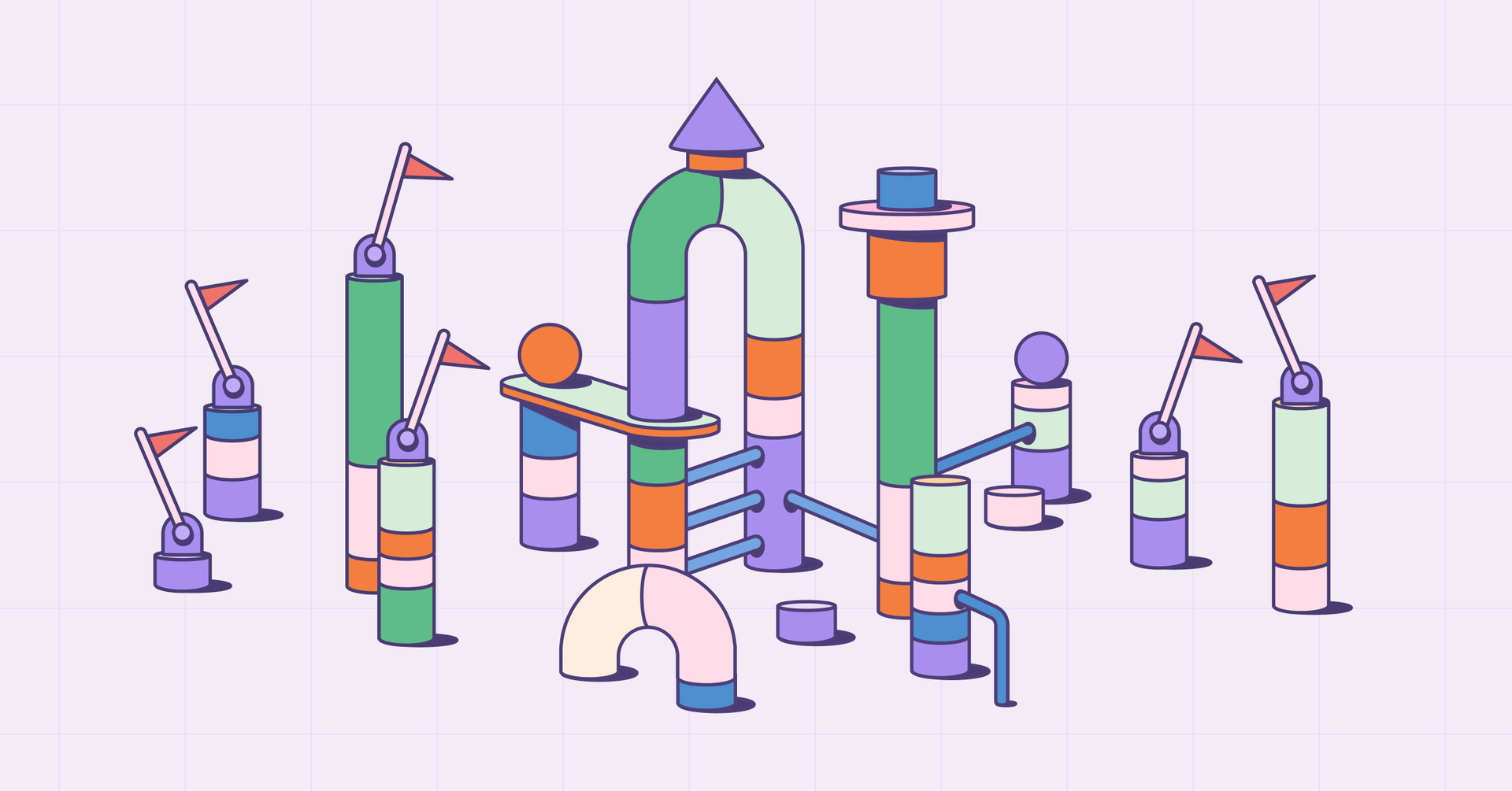
AI copilots: challenges and solutions
1. Privacy and security concerns
The challenge:
Privacy and security are critical for any organization. AI copilots, which require access to sensitive data, raise security concerns, which in turn can slow down adoption. According to a recent report by Tines, 66% of CISOs consider data privacy a challenge to AI adoption.
The solution:
AI tools need to be supported by robust privacy and security features. The user’s data shouldn’t leave the stack, travel on the internet, be logged, or be used for training. Enterprise-grade controls are essential, and should include role-based access, confirmation messages, and audit logs.
2. Lack of secure access to proprietary data
The challenge:
AI copilots require access to data from across the company’s systems in order to function, but security concerns can make this difficult, reducing the copilot’s effectiveness.
The solution:
The ideal AI chat interface provides users with access to proprietary data in real-time, enhancing decision-making. This can only be done when appropriate security and privacy guardrails are in place, and when AI can easily connect to the relevant tools in the stack.
3. AI can’t take action on your behalf
The challenge:
One of the big promises made by many AI copilots is that they can take action, but there may be unexpected challenges if they only work with a specific set of tools.
The solution:
Teams need an AI chat interface that can take action through workflow automation - of course, only when authorized users instruct it to do so. This would help enterprises reduce response times and improve overall efficiency.
4. Siloed to specific products
The challenge:
Data is often scattered across many systems - the average security team has access to 76 tools. If a copilot can’t connect to all of them, multiple AI solutions may be required, which is costly and threatens to increase response times.
The solution:
An ideal AI product integrates with any technology via API - users define the tools it can access and the specific actions it can perform within each tool.
5. Lack of visibility or choice on LLMs provided
The challenge:
Users sometimes lack visibility into which large language model (LLM) their AI copilot uses, which can introduce additional security and privacy risks.
The solution:
AI chat interfaces should clearly indicate which LLM they use. If there are options available, this information should also be communicated.
3 things to look for in AI tools
To ensure AI has a worthwhile impact on an organization’s growth, teams should prioritize:
- Security and privacy - security features that protect sensitive data
- Interoperability - hassle-free integration with existing systems
- Ability to act - the capability to take action when authorized by an approved user
One of the first AI products to satisfy all these criteria is Tines Workbench.
Built on the same secure infrastructure of the Tines automation and orchestration platform, Workbench puts users in control - they determine what AI can and cannot do.
After connecting Workbench to any tool in their stack, users can give it permission to perform tasks like sending a message in Slack, looking up an employee in an HRIS, creating a ticket in Jira, getting detections in CrowdStrike Falcon, searching Elastic for alerts based on specific criteria or locking down a device in Jamf.
The AI copilot landscape is evolving rapidly, but recognizing the features that drive meaningful impact is the crucial first step to unlocking its full potential.
Learn how Tines Workbench helps security teams work and respond faster.
Author: Eoin Hinchy